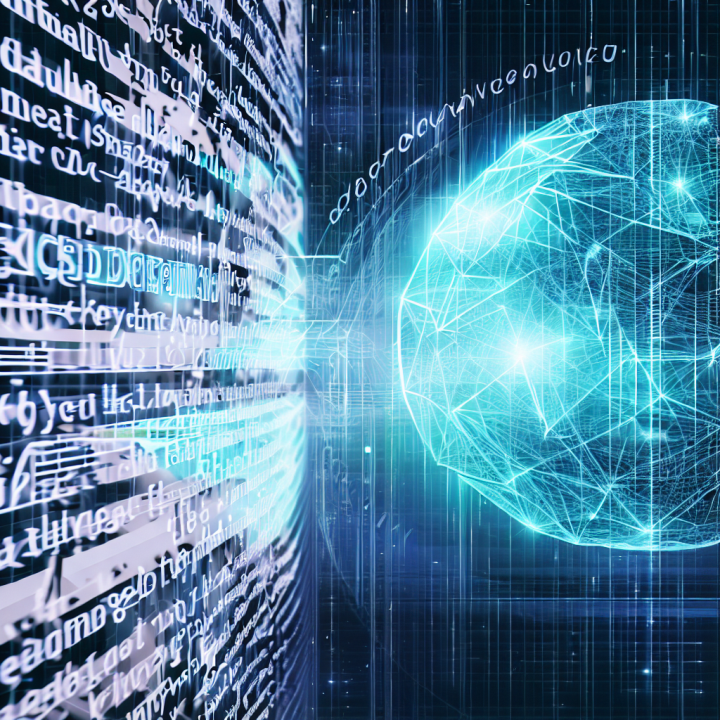
Research overview
Research projects in this theme involve the use of knowledge graphs (including our EpiGraphDB), language models such as Google’s BERT and large language models (LLMS) such as GPT-4, Llama and Falcon.
Applications of knowledge graphs
We are interested in the use of knowledge graphs to identify new hypotheses and triangulate information. An example of this is work led by PhD student Marina Vabistsevits on the identification of risk factors for breast cancer. This work involved the integration of evidence from Mendelian randomization causal estimates and literature triples, both derived from EpiGraphDB.
Link prediction in knowledge graphs
Knowledge graph embedding methods offer the potential to identify errors in knowledge graphs, and potentially to generate new hypotheses. In work led by PhD student Ollie Lloyd, we have evaluated the effects of hyperparameters on knowledge graph embeddings using a range of different methods, and are now applying these to predict polypharmacy side-effects.
Natural language interfaces to knowledge graphs
New language models offer the opportunity to create natural language interfaces to databases. In work led by Research Fellow Yi Liu, we implemented the Annotated Semantic Queries (ASQ) platform that provides a natural language query interface to the integrated biomedical entities and epidemiological evidence in EpiGraphDB.
Using language models to automate systematic reviews
In work funded by the World Cancer Research Fund, and led by Research Fellow Yi Liu, Senior Research Associate Maria Sobczyk-Barad and Senior Research Associate Zhaozheng Xu, we are using various language models to automate aspects of the systematic review process.